Drug development has been plummeting in productivity over the last 70 years. Learn how improving preclinical models is the key to solving the challenge at the heart of drug development.
“The more positive anyone is about the past several decades of progress [in pharmaceutical development], the more negative they should be about the strength of countervailing forces.” These foreboding words were penned in a seminal 2012 article by Jack Scannell, author of Eroom’s Law, in an effort to illuminate the drug development industry’s productivity crisis.
Productivity measures how efficient drug development is, often presented as the number of drugs that can be brought to market given a set amount of effort or investment. Consider pharmaceutical development in the 1950s, for example: Data presented by Scannell and his co-authors showed that, with the contemporary equivalent of $1 billion US dollars, the industry was able to produce around 30 new drugs. In contrast, that same investment of $1 billion in 2023 would not even produce one new therapeutic (see Figure 1).
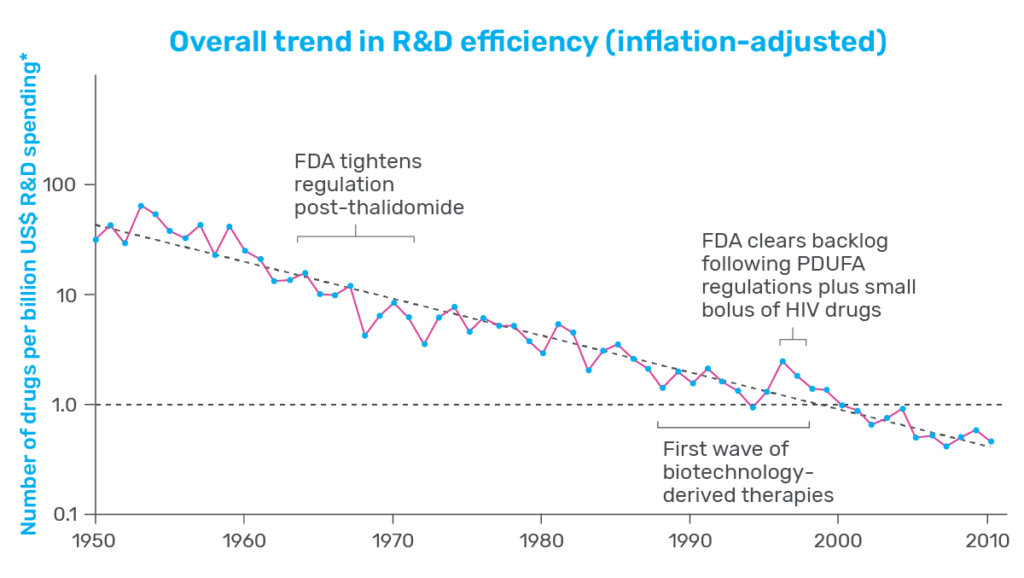
That is a substantial decrease in productivity and one that many in the industry are concerned about. Whether it’s to treat neurodegenerative disease, stem the spread of infectious agents, or fight cancer, there is a persistent need among patients for new and innovative therapeutics. When productivity is low, developers face steeper costs, and progress slows. As a result, drug costs increase for patients, who are left waiting in desperate need of therapeutic relief.
Diagnosing the various factors that have led to our current productivity crisis—the “countervailing forces”—has been a challenge that Scannell and many others have worked to overcome. Through their efforts, many potential causes have been identified, one of which stands out as profoundly impactful: improving the accuracy of preclinical models.
Preclinical Drug Development
Preclinical drug development is highly speculative: Researchers are tasked with foretelling how compounds will behave in the human body and ultimately identifying the select few that are both safe and therapeutic. To do this, they rely on model systems that serve as proxies for the human body, with none serving a more prominent role than non-human animal models.
Rodents, primates, and other non-human animal models have long been the gold standard in preclinical toxicology screening. With complex and interconnected tissues, these animals allow researchers to test the effect of their drug in a dynamic system that resembles the human body. As such, animals have been positioned as the last filter in the drug development process, charged with the difficult job of weeding out toxic drugs before they enter clinical trials.
Despite their ubiquity in drug development, ample evidence indicates that animal models are far from perfect. Approximately 90% of drugs entering clinical trials fail, with roughly 30% of those failures attributed to unforeseen toxicity. Such abundant failure indicates that, at minimum, animal models alone are insufficient decision-making tools—too often, they get it wrong, and both patients and drug developers pay the price.
The cost of this failure plays a central role in the current productivity crisis. Though researchers now have access to next-generation sequencing, combinatorial chemistry, and automation, drug development costs have increased nearly 80-fold since 1950 to a staggering $2.3B per approved drug. And approximately 75% of the pharmaceutical industry’s drug development costs can be attributed to development failures.
It stands to reason that reducing clinical trial failure rates will improve the efficiency of drug development. Not only are failed trials expensive, but they also take up clinical resources that could otherwise be used to advance successful drugs. Since clinical trial failure rates reflect the quality of drugs that enter trials, improving the quality of these drugs should improve the industry’s overall productivity.
So how should researchers go about doing this? Revisiting his seminal work a decade later, Scannell provided powerful guidance: The quality of the compounds that enter clinical trials is a consequence of the preclinical models used to select them, and even small improvement in the quality of the preclinical models—more specifically, their predictive validity—can have a substantial impact on productivity. Enter more human-relevant preclinical models like the Liver-Chip.
Improving Productivity with Organ-Chips
In a recently published study, Emulate scientists showed that the Liver-Chip—a specialized Organ-Chip that mimics the human liver—can identify compounds’ potential to cause drug-induced liver injury (DILI) far more accurately than traditional in vitro and animal models.
Briefly, Organ-Chips are three-dimensional culture systems that combine heterogeneous cell culture, fluid flow, and several features of the tissue microenvironment to mimic human organ function in an in vitro setting. Evidence indicates that human cells cultured in Organ-Chips behave remarkably similar to their in vivo counterparts. Among many promising applications, these chips are particularly well suited for preclinical toxicology screening.
In their study, the Emulate researchers found the Liver-Chip to be a highly sensitive and specific tool for detecting hepatotoxic compounds. In particular, the Liver-Chip showed a sensitivity of 87% and specificity of 100% against a series of drugs that had progressed into the clinic after being tested in animal models, only to later be revealed as toxic when given to patients. Therefore, these drugs well represent the current gap in preclinical toxicology testing, through which some hepatotoxic drug candidates evade detection and advance into clinical trials.
If the Liver-Chip can fill the gap left by animal models, Scannell’s framework suggests that the Liver-Chip could profoundly affect the industry’s productivity by reducing the number of safety-related clinical trial failures.
To calculate how this reduction may impact industry productivity, Emulate researchers teamed up with Jack Scannell to build an economic value model. This analysis showed that applying the Liver-Chip in all small-molecule drug development programs could generate $3 billion dollars annually for the industry as a result of improved productivity. This is approximately $150M per top pharmaceutical company. And, that’s just for the Liver-Chip. In addition to hepatotoxicity, cardiovascular, neurological, immunological, and gastrointestinal toxicities are among the most common reasons clinical trials fail. If Organ-Chips can be developed to reduce these clinical trial failures with a similar 87% sensitivity, the resulting uplift in productivity could generate $24 billion for the industry annually—roughly $750M to $1B per top pharmaceutical company.
It is immediately evident that, even when the cost of integrating and running Liver-Chip experiments is accounted for, the cost savings of reducing clinical trial failures is substantial. Moreover, the freed-up clinical bandwidth would permit advancing other, more promising compounds. The Emulate researchers’ work demonstrates that improving productivity in drug development is possible, and it starts with developing better models. As the industry embraces the potential of Organ-on-a-Chip technology and continues to explore its application in various areas of drug development, there is hope for a future with improved productivity and faster delivery of life-saving therapeutics.